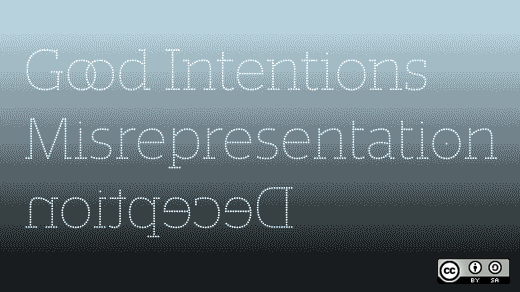
Computer science as a field lacks definite supportive principles about “social good”. Instead, the field relies on vague propositions and aspects of social conditions that do not amount to the actual social change. This has continuously hindered the realization of social impact through technological interventions in various sectors and levels including commercial, non-commercial, and government. The field lacks well-developed methods and principles for understanding the relationship between technological interventions and social impact. As a result, the efforts aimed at achieving social good fail to realize substantive social change.
This paper evaluates the phrase “social good” in the field of computer science including its various interpretations and implementations. The author analyzes different political and normative proxies of “social good” and their adoption in the field. It discusses how these interpretations influence technological interventions which in turn result in unexpected outcomes in terms of impacting social change. The author concludes by proposing rational strategies and methods for implementing information technologies in ways that can result in positive social impact.
Other than Mechanism Design for Social Good, established in 2017, the field of computer science lacks a working definition of “social good”. Surprisingly, there are no definitions of “social good” in several dictionaries except in Investopedia, a financial education website where it is defined as “something that benefits the largest number of people in the largest possible way, such as clean air, clean water, health and literacy.” Despite the lack of grounding principles, many computer scientists have worked on projects that claim to promote “social good” in conflicting ways which raises the question as to what the projects stand for. The author argues that while there is no optimal definition of “social good”, there should be frameworks for debates and perspectives on what good actually entails.
The author gives an example of USC’s Center for Artificial Intelligence in Society (CAIS), a body responsible for promoting “social good”, but instead causes harm through its regressive approach. In one of its projects aimed to predicting and preventing behavior from adversarial groups, it instead promotes an old policing system by classifying criminal street gangs and terrorists as “superpredators”, a strategy used to target poor and minority neighborhoods in Los Angeles Police Department. Although such projects are intended to promote social good, they only represent a portion of the society, typically, the dominant social groups hence not beneficial to all. Therefore, the field of computer science needs to openly reflect on the assumptions and values underlying its key aspects in order to expel the structural social oppressions as a way of achieving “social good”.
Algorithmic interventions and machine learning, in particular, can result in short-term achievements such as being able to predict the behavior of adversarial groups. However, computer scientists should not focus on the immediate achievements at the expense of long-term impacts that may cause great harm. The same algorithms can be used to better many aspects of society. Moreover, the incremental goods obtained through algorithmic interventions should be evaluated depending on the type of society it promotes both in short and long term.
To understand the relationship between the incremental goods and the long-term social impacts, the author adopts Andre Gorz’s two types of reforms: reformist reforms and non-reformist reforms. According to Gorz, a reformist reform follows the rationality and applicability of a given system while a non-reformist reform relies on what’s possible based on human needs and demands. Most computer science interventions are reformist reforms that tend to improve the performance of a system rather than alter it. As a result, they are limited to narrow parameters of change rather than focusing on the wider social and political spectrum. The author compares this type of reform to a greedy algorithm useful only for simple problems but, cannot be accepted for modeling complex optimization problems.
To expound on the limitations of reformist reforms, the author highlights the dangers of “good” reforms in the U.S. criminal justice system. Despite the efforts of computer scientists to reform the criminal justice system, they tend to reproduce and reinforce the existing structural racial violence in the system. Notably, such reforms should be evaluated based on a broader vision of long-term changes.
In conclusion, the author reiterates that the field of computer science must develop a rigorous methodology for understanding the actual good and how to choose from competing goods. This calls for political orientation of algorithmic practice and explicit use of social good in computer science projects and interventions. Computer scientists should contextually understand the relationship between technological interventions and social impact in both the long and short term. Finally, the author proposes that computer scientists should explore alternative reforms such as algorithmic interventions that ensure long-term achievement of social change.
Summary by Eugene Oduma